In recent years, geostatistics has assumed a key role in modeling and predicting geophysical variables. The main goal of geostatistics is to provide methodologies able to describe and forecast quantitative variables distributed over space, or space-time.The focus of the project is posed on the geostatistical modeling and prediction of complex geophysical variables, such as high-dimensional, constrained or functional data. Our unifying idea when dealing with these different types of data is to consider each datum as an object of an appropriate space (finite- or infinite-dimensional), and consistently define spatial dependence through a geometric approach [1].Results attained within the project include the extension of spatial prediction techniques (e.g., kriging) to either stationary or non-stationary Hilbert-space valued random fields [1]. This general theoretical framework enables one to treat data-objects of several types, such as multivariate, functional, or compositional data, within a unitary setting.
An example of application-oriented problem addressed on these bases concerns the geostatistical characterization of particle-size distributions within a heterogeneous aquifer ([2,3] and Fig. 1). Indeed, even particle-size distributions can be treated within the framework of object oriented geostatistics, by analyzing their densities via the Aitchison geometry for functional compositions. Characterizing the spatial distribution of particle-size data is relevant to properly reconstruct the internal architecture of groundwater systems, which in turn can play a critical role, e.g., in controlling contaminant spreading on different scales.
References
[1] Menafoglio, A., P. Secchi, and M. Dalla Rosa (2013). A Universal Kriging predictor for spatially dependent functional data of a Hilbert Space. Electronic Journal of Statistics 7, 2209–2240.[2] Menafoglio, A., A. Guadagnini, and P. Secchi (2014a). A Kriging Approach based on Aitchison Geometry for the Characterization of Particle-Size Curves in Heterogeneous Aquifers. Stoch. Env. Res. Risk A. 28(7), 1835-1851[3] Menafoglio, A., P. Secchi and A. Guadagnini (2014b). A Class-Kriging predictor for Functional Compositions with Application to Particle-Size Curves in Heterogeneous Aquifers. MOX-report.
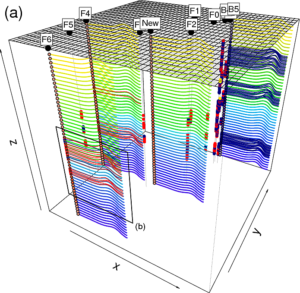
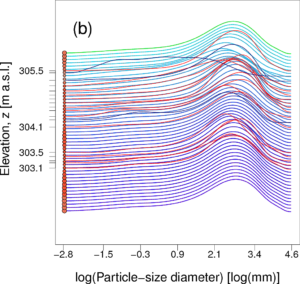
Application of object-oriented geostatistics to a field study: geostatistical characterization of particle-size distributions in a heterogeneous aquifer system [2,3]. The Kriging prediction is performed on the basis of 406 particle-size densities (only partially reported), available at 12 boreholes. Colors of the symbols represent the soil type associated with the PSDs, which is properly taken into account while performing Kriging [3].