Advancing Scientific Machine Learning in Industry
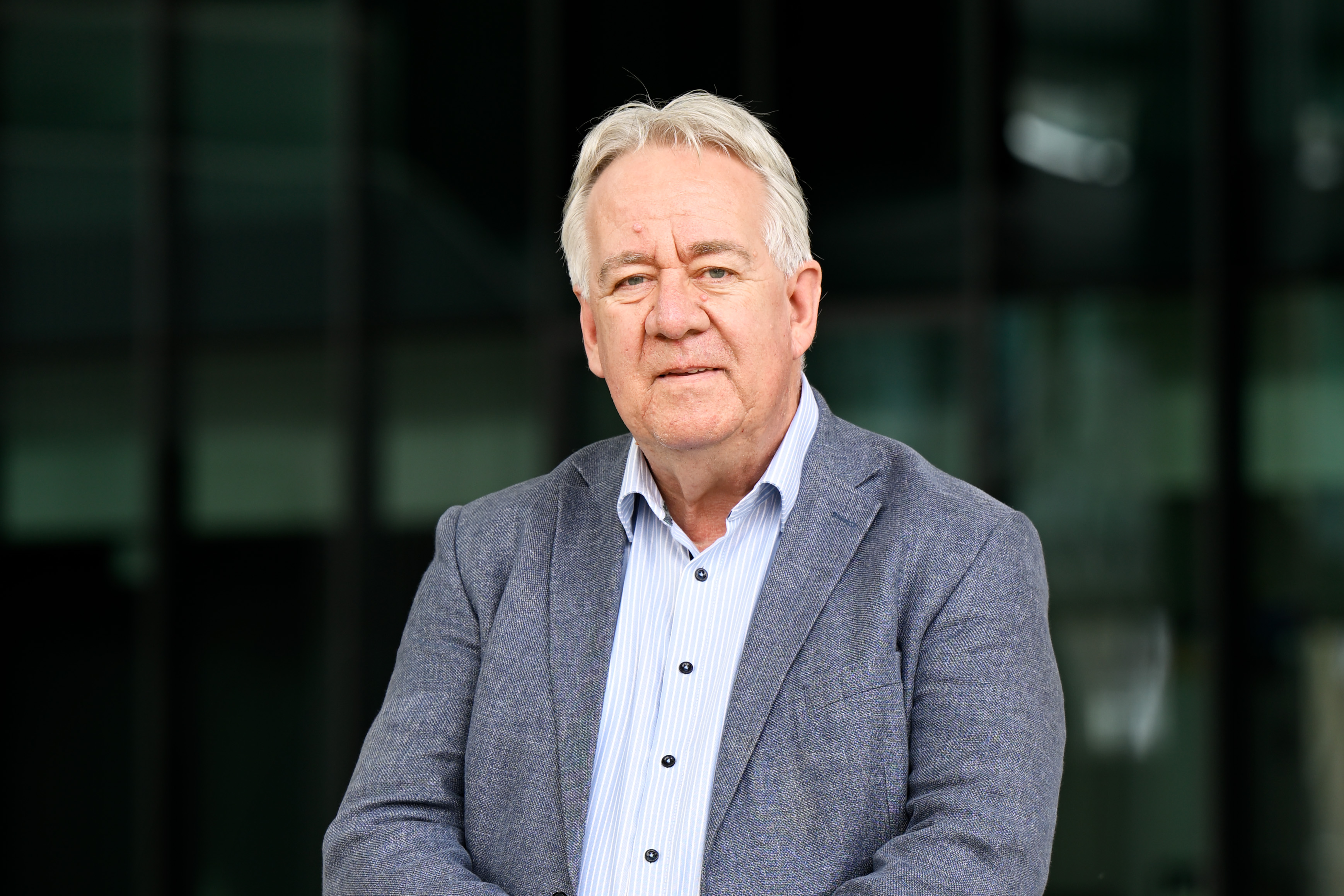
Keywords
Speaker:
Wil Schilders
Affiliation:
Eindhoven University of Technology and TU Munich-Institute for Advanced Study
When:
Wednesday 26th March 2025
Time:
14:00:00
Where:
Aula Castigliano, Politecnico di Milano
Link to seminar:
Link to seminar will become active ten minutes before due starting time: Click HERE to refresh page
Abstract:
Scientific machine learning (SciML) has been taking the academic world by storm as an interesting blend of traditional scientific modeling with machine learning (ML) methodologies like deep learning. While traditional machine learning methodologies have difficulties with scientific issues like interpretability, and enforcing physical constraints, the blend of ML with numerical analysis and differential equations has evolved into a novel field of research which overcome these problems while adding the data-driven automatic learning features of modern machine learning. Many successes have already been demonstrated, with tools like physics-informed neural networks, universal differential equations, deep backward stochastic differential equation solvers for high dimensional partial differential equations, and neural surrogates showcasing how deep learning can greatly improve scientific modeling practice. Consequently, SciML holds promise for versatile application across a wide spectrum of scientific disciplines, ranging from the investigation of subatomic particles to the comprehension of macroscopic systems like economies and climates.
However, despite notable strides in enhancing the speed and accuracy of these methodologies, their utility in practical and specifically industrial settings remain constrained. Many domains within the scientific community still lack comprehensive validation and robustness testing of SciML approaches. This limitation is particularly pronounced when confronted with complex, real-world datasets emanating from interactions between machinery and environmental sensors as usually addressed in industry. Still if appropriately addressed, SciML with its promise to accelerate innovations and scientific discoveries by orders of magnitudes, offers unique opportunities to address the insatiable desire for faster and more accurate predictions in many fields.
This presentation is dedicated to exploring recent advancements in the implementation of SciML techniques. We will discuss how methodologies can be refined to ensure their practical viability and scalability, particularly in industrial sectors where digital and physical components converge.
Contatto:
paola.antonietti@polimi.it
Note:
Wil Schilders studied Mathematics, with Physics and Astronomy, at the Radboud University in Nijmegen, The Netherlands, from 1974-1978, and obtained his PhD in numerical analysis from Trinity College Dublin in 1980. From 1980-2006 he worked at Philips Research, performing research on mathematical methods and software for semiconductor device simulation and electronic circuit simulation, and from 2006-2010 at NXP Semiconductors. Since 1999, he was also a part-time professor at TU Eindhoven on scientific computing for industry. In 2010, he moved to TU Eindhoven, and also became the director of the Dutch Platform for Mathematics. He has been active within the European Consortium for Mathematics in Industry (ECMI), being president in 2010-2011 and chairing the Research and Innovation Committee for many years. From 2015-20, he was president of EU-MATHS-IN, the European Service Network of Mathematics for Industry and Innovation (www.eu-maths-in.eu). Since October 1, 2023, he is the president of the International Council for Industrial and Applied Mathematics (ICIAM). In 2020, he was the 4th Mittelsten-Scheid guest professor at Bergische Universitaet Wuppertal, and since 2021 he is a Hans Fischer Senior Fellow at the Institute for Advanced Study at TU Munich. He is a fellow of the Society for Industrial and Applied Mathematics (SIAM) and was awarded the Dutch Stairway to Impact award end of 2022. In 2023, he organized the SIAM conference on Computational Science and Engineering in Amsterdam, with 2100+ participants. He supervised 25 PhD students, and led more than 10 European projects. His main expertise is in numerical linear algebra, model order reduction (he was chair of EU-MORNET) and recently he has become interested in scientific machine learning, a combination of numerical analysis/scientific computing and machine learning (his motto: ‘’Real intelligence is needed to make artificial intelligence work’’). He has a large network, also with industry, and is very interested in sustainability and the role of mathematics herein.