Learning physics-based models from data:
Perspectives from model reduction
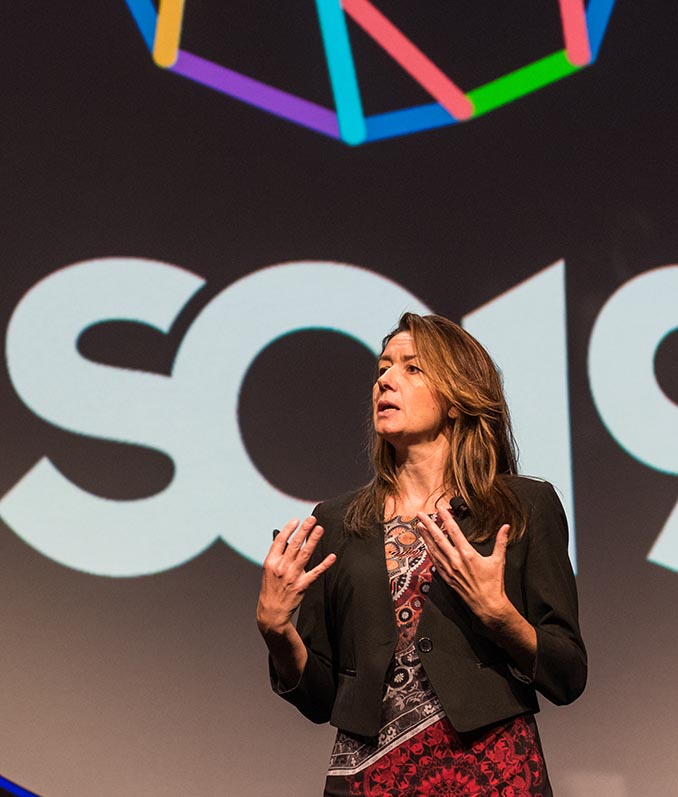
Keywords
Advanced Numerical Methods for Scientific Computing
Speaker:
Karen E. Willcox
Affiliation:
University of Texas at Austin
When:
Thursday 24th February 2022
Time:
15:00:00
Where:
Online seminar: https://mox.polimi.it/mox-seminars/?id_evento=2132
Link to recording:
Abstract:
Operator Inference is a method for learning predictive reduced-order models from data. The method targets the derivation of a reduced-order model of an expensive high-fidelity simulator that solves known governing equations. Rather than learn a generic approximation with weak enforcement of the physics, we learn low-dimensional operators whose structure is defined by the physical problem being modeled. These reduced operators are determined by solving a linear least squares problem, making Operator Inference scalable to high-dimensional problems. The method is entirely non-intrusive, meaning that it requires simulation snapshot data but does not require access to or modification of the high-fidelity source code. For problems where the complexity of the physics does not admit a global low-rank structure, we construct a nonlinear approximation space. This is achieved via clustering to obtain localized Operator Inference models. The methodology is demonstrated on challenging large-scale problems arising in rocket combustion and materials phase-field applications. Joint work with Rudy Geelen.
Note:
Karen E. Willcox is Director of the Oden Institute for Computational Engineering and Sciences, Associate Vice President for Research, and Professor of Aerospace Engineering and Engineering Mechanics at the University of Texas at Austin. She holds the W. A. “Tex” Moncrief, Jr. Chair in Simulation-Based Engineering and Sciences and the Peter O'Donnell, Jr. Centennial Chair in Computing Systems. Prior to joining the Oden Institute in 2018, she spent 17 years as a professor at the Massachusetts Institute of Technology, where she served as Professor of Aeronautics and Astronautics, the founding Co-Director of the MIT Center for Computational Engineering, and the Associate Head of the MIT Department of Aeronautics and Astronautics. She is also an External Professor at the Santa Fe Institute.
Willcox holds a Bachelor of Engineering Degree from the University of Auckland, New Zealand, and masters and PhD degrees from MIT. Prior to becoming a professor at MIT, she worked at Boeing Phantom Works with the Blended-Wing-Body aircraft design group. Her research has produced scalable computational methods for design of next-generation engineered systems, with a particular focus on reduced-order modeling as a way to learn principled physics-based approximations from data and on multifidelity formulations to leverage multiple sources of information in decision-making and uncertainty quantification. Her model reduction and multifidelity methods are widely applied across the scientific and engineering community, and have been incorporated into industry/government codes for aircraft system design and environmental policy decision-making. She currently has funded projects supported by the US Air Force Office of Scientific Research, Air Force Research Laboratory, ARPA-E, Department of Energy, Lockheed Martin, NASA, and Sandia National Laboratories. Willcox currently leads several multi-institution research teams: she is Co-director of the Department of Energy AEOLUS Multifaceted Mathematics Capability Center on Advances in Experimental Design, Optimal Control, and Learning for Uncertain Complex Systems; she leads an Air Force MURI team on Machine Learning for Physics-based Systems; and she leads the Rise of the Machines team developing robust, interpretable, scalable, efficient methods for digital twins under the Department of Energy AI and Decision Support for Complex Systems program. Willcox has co-authored more than 120 papers in peer-reviewed journals and advised 62 graduate students, including 21 PhD students.
In addition to her research pursuits, Willcox is active in education innovation. She served as co-Chair of the MIT Online Education Policy Initiative, co-Chair of the 2013-2014 Institute wide Task Force on the Future of MIT Education, and Chair of the MIT OpenCourseWare Faculty Advisory Board. She is a recognized innovator in the U.S. education landscape, where she is a 2015 recipient of a First in the World Department of Education grant that developed and deployed educational technologies in community colleges. She continues to direct the MIT Mapping Lab, which develops technologies for the future of digital education.
Willcox is Fellow of the Society for Industrial and Applied Mathematics (SIAM), Fellow of the American Institute of Aeronautics and Astronautics (AIAA), and member of the American Society for Engineering Education (ASEE). She has served in multiple leadership positions within AIAA and SIAM, including leadership roles in the SIAM Activity Group on Computational Science and Engineering, the SIAM Activity Group on Data Science, and the AIAA Multidisciplinary Design Optimization Technical Committee. She is currently a member of the AIAA Board of Trustees. She is formerly Section Editor of SIAM Journal on Scientific Computing and current Editorial Board member of AIAA Journal. She is a current member of the National Academies Board on Mathematical Sciences and Analytics, and has served on five National Academies studies and review panels. In 2017, she was awarded Member of the New Zealand Order of Merit (MNZM) for services to aerospace engineering and education.